Laboratory of Systems Biology
Vision
Despite our rapidly improving ability to
probe molecular and cellular states, our understanding of biology
remains largely descriptive and often overwhelmed by details of
unclear significance. Our research is driven by the desire to move
beyond the vast scale of these detailed descriptions to discover the
underlying organizing principles. These principles reflect
fundamental solutions to common challenges revealed by natural
selection across billions of years of evolution. In essence, these
principles constitute a compact set of concepts and algorithms that
enable us to broadly generalize our understanding of biological
phenomena, to efficiently manipulate them for medical and industrial
purposes, and to engineer biological systems from first principles.
As such, our research is focused on systems-level phenomena
independent of conventionally defined processes and pathways. We
employ diverse experimental systems from bacteria to mammalian
cell-lines in order to study general principles that operate across
organismal taxa and complexity. Our
systems-level studies often necessitate observations,
perturbations, or analyses that are beyond the scale and
precision of existing methods. We thus develop new enabling
technologies and computational methods that significantly
advance our ability to study systems-level phenomena.
Approach
Our vision of systems biology originated
when the very first microarray gene expression datasets appeared in
the late 1990’s. At the time, we wondered whether such large-scale
observations, coupled with properly constrained machine-learning,
would enable us to discover the inherent organization of gene
regulatory networks. Our work demonstrated that, indeed, this
strategy can systematically reveal the regulatory vocabulary and
grammar in DNA, enabling prediction of global gene expression
dynamics from DNA sequence alone. The ability to rapidly rediscover
gene regulatory programs that had taken decades to uncover
demonstrated the power of this ‘reverse engineering’ paradigm for
rapidly advancing biology. Over the years, we have established the
unique efficacy of this paradigm across diverse domains of biology
including transcriptional & post-transcriptional regulation,
chromatin modification dynamics, molecular interaction mapping,
fitness landscape characterization, genetic interaction mapping, and
genotype-phenotype inference. These studies have revealed
fundamental new insights into the genetic and regulatory
underpinnings of a variety of phenomena including complex bacterial
behaviors, antibiotic resistance & persistence, metazoan
development, oncogenesis, metastatic progression, and cellular
adaptation to extreme environments. Many of the approaches and
technologies we have introduced have made broad impact through their
adoption across diverse areas of biology.
Gene regulation
Context-dependent regulation of gene
expression is fundamental to all cellular behaviors. The expression
of a gene is shaped by the convergence of upstream inputs that
impinge upon DNA and RNA sequence elements in the vicinity of genes,
leading to precise modulation of global mRNA and protein abundances.
The richness of gene expression programs—in a given cell across
time, across distinct cell types, and in response to diverse
stimuli—results from the combinatorial logic of spatially organized
nucleic acid elements that bind transcription factors, RNA binding
proteins, and microRNAs. The identification of these regulatory
elements and elucidation of the rules by which they operate remains
a central challenge for modern biology. With the arrival of the very
first microarray expression experiments, we reasoned that the
massive scale of the data may enable an entirely agnostic approach
to discovering the underlying regulatory logic of gene expression.
We thus applied unsupervised machine learning approaches to first
discover the intrinsic patterns of gene expression (clusters), and
then used ab initio motif discovery to reveal highly
enriched DNA-sequence motifs, representing transcription factor
binding sites through which gene expression is modulated. We showed
that this agnostic strategy can systematically reveal the known
transcriptional regulatory architecture of the yeast cell-cycle and
predict novel regulators that have since been discovered by us and
others (Tavazoie et al., Nature Genetics, 1999).
We also introduced pathway enrichment analysis that has
since become a critical tool for interpreting the biological
significance of genome-wide studies.
Motivated by the desire to build predictive
models of gene expression, we used supervised machine learning
approaches to learn the combinatorial grammar in regulatory regions,
revealing the need for precise spatial configuration of multiple
transcription factor binding sites. We showed that, in yeast, these
models can achieve surprisingly high performance, enabling
prediction of global gene expression dynamics directly from DNA
sequence alone (Beer & Tavazoie,
Cell, 2004). We developed next-generation reverse engineering approaches
based on information-theory that reveal transcriptional and
post-transcriptional regulatory elements with high sensitivity and
specificity in organisms ranging from bacteria to human (Elemento et
al., Molecular Cell, 2007; Goodarzi et al., Molecular
Cell, 2009). The incorporation of complex representations of sequence and
structure allowed us to discover a large regulatory landscape of
post-transcriptional regulation by RNA-structural elements in 5’ and
3’ non-coding regions of mRNAs (Goodarzi et al., Nature,
2012).
We
showed that these regulatory elements and the RNA binding proteins
that bind them regulate critical pathways in physiology and disease,
including cancer metastasis (Goodarzi et al., Nature,
2014).
We
are currently using these computational tools, together with
CRISPR-based functional profiling technologies, to map the
post-transcriptional regulatory landscape of oncogenesis and cancer
metastasis. Another area of interest is the application of these
approaches to decode the transcriptional and post-transcriptional
regulatory networks critical for differentiation and development,
including those that establish identity and function in the nervous
system (Taylor et al., Cell, 2021).
Modern deep learning architectures are an increasingly important
tool for building predictive models that reveal the key regulatory
parameters in the central dogma including those modulating
transcription, mRNA stability, translation, post-translational
modifications, localization, and protein stability.
Cellular adaptation
A major focus of our work is to understand
how cells adapt to changes in their external environment. We study
this inherently systems-level phenomenon across a range of
timescales, from rapid transcriptional responses, to
multi-generational epigenetic reprogramming, to long-term rewiring
of signaling and regulatory networks over evolutionary timescales.
Much of our contributions have resulted from questioning long
prevailing dogma in the field. By posing problems as open questions,
the agnostic nature of our approach allows the system itself to
reveal the essential governing principles. This has been crucial to
discovering surprising new phenomena such as the ability of microbes
to carry out predictive behavior akin to metazoan nervous systems (Tagkopoulos
et al. Science, 2008). Often these higher-level principles are obscured by approaches
that focus only on a narrow slice of the cell's response.
By challenging fundamental notions of
cellular adaptation, we have found that eukaryotic cells utilize a
noise-driven optimization mechanism to reprogram gene expression in
a manner that is independent of conventional gene regulation. In
this process that we call stochastic
tuning, cells utilize the inherent noise in mRNA transcription
to randomly increase or decrease expression of genes and to actively
reinforce changes that improve the overall health of the cell. We
have shown that this mechanism enables cells to empirically
establish adaptive gene expression levels in the absence of
conventional hard-wired regulatory input (Freddolino et al., eLife
2018).
A
such, stochastic tuning is an active form of gene expression
optimization that occurs in individual cells—a phenomenon distinct
from population-level bet-hedging. We have shown that stochastic
tuning occurs in budding yeast, enabling cells to adapt to
unfamiliar environments where their conventional regulatory systems
are challenged beyond their physiological operating capacity. Since
the pioneering work of Jacob and Monod sixty years ago, we have been
taught that cellular responses are determined by pre-defined
regulatory programs established by specific genetically encoded
pathways (e.g. the
osmolarity response pathway). The fact that individual cells can,
instead, utilize a noise-driven trial & error process to empirically
establish gene expression levels goes against our most cherished
notions of gene regulation and cellular adaptation. Beyond its role
in adaptation of single-cell eukaryotes, stochastic tuning may be
the underlying mechanism for observed non-mutational tumor
adaptation, increasingly recognized as a key factor in chemotherapy
failure. Identifying the molecular components of stochastic tuning,
discovering the detailed underlying mechanisms, and exploring the
breadth of its pathophysiological significance are major areas of
focus for current and future work in the lab.
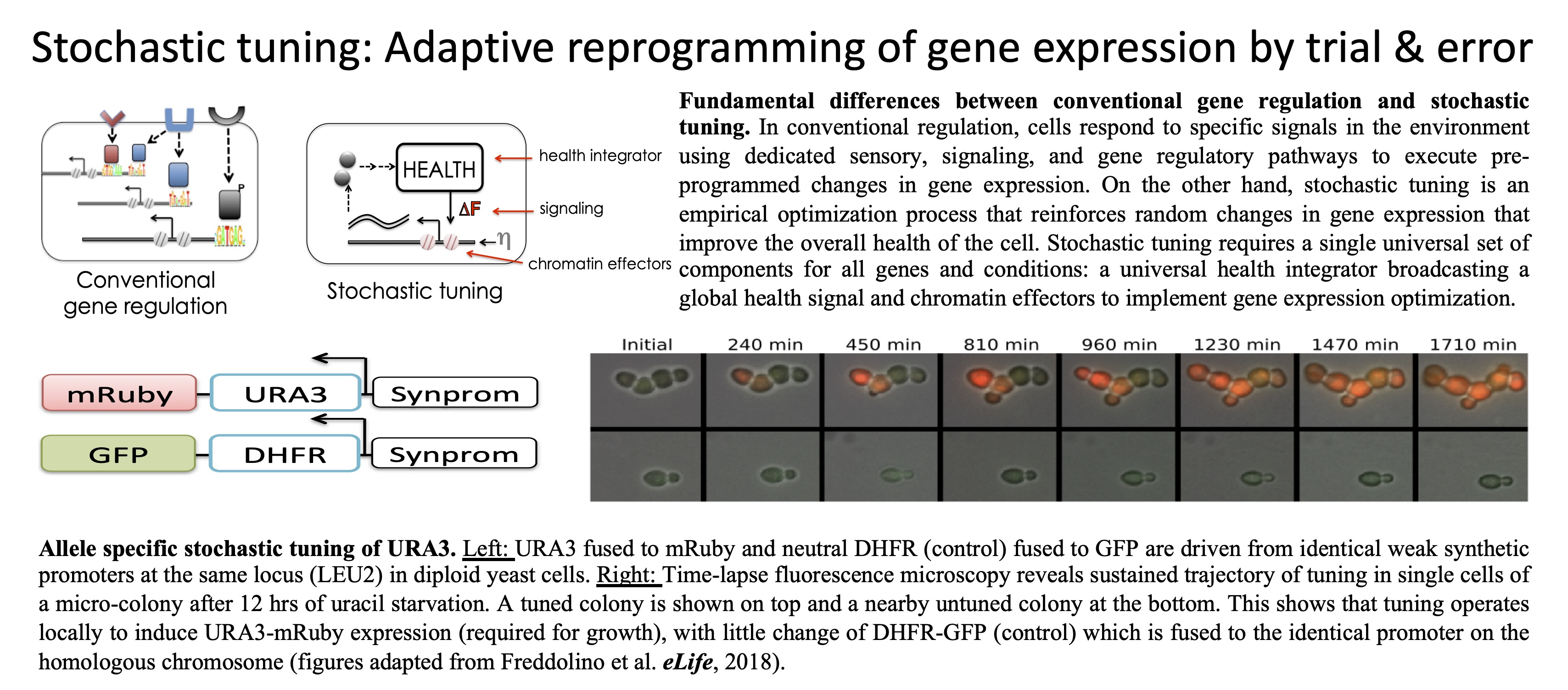
Molecular interactions
Molecular interactions are the fundamental
building blocks of life. Almost every biological process can be
understood in terms of specific interactions between key
biomolecules such as proteins, DNA, RNA, and metabolites. Mapping
and understanding the astronomically complex network of molecular
interactions among the millions of distinct components in the cell
remains a grand challenge with far-reaching implications across all
areas of biology. For example, if we focus on the human proteome
(even ignoring differential splice variants), there are some ~109
possible pair-wise interactions. This number grows to ~1012 for
all potential human DNA-protein interactions at 15 bp resolution. In
a perfect world, a technology would allow us to efficiently,
systematically, and quantitatively, measure all these
potential interactions, providing a global unbiased architectural
view. The advent of yeast two-hybrid technology (Y2H) more than two
decades ago, initiated an effort to move in this direction and
indeed the emerging maps have formed a critical foundation for
modern biology. However, the sensitivity, cost, and labor-intensive
nature of Y2H and many alternative variants do not permit routine
mapping and monitoring of global molecular interactions as a
function of varying molecular and cellular states in vivo.
We are developing next-generation technologies to address these
limitations.
A major focus has been to monitor in
vivo protein-DNA interactions as a function of changing
conditions. We have developed a biochemical procedure to efficiently
isolate protein bound sites throughout a bacterial genome and to
quantify their occupancy using next-generation sequencing. This
approach, called IPOD (In vivo Protein Occupancy
Display) enables comprehensive global monitoring of
protein-DNA interactions as a function of genetic and environmental
perturbations (Vora et al., Molecular
Cell, 2009). These dynamic global maps can be readily utilized to identify
specific genes and regulatory regions that are dynamically activated
as a function of any perturbation, revealing the vast regulatory
landscape that is obscured when the genome is only interrogated
through single ChIP-seq experiments (Freddolino et al., PLoS Biology, 2021).
The ability to globally monitor all such sites, without prior bias,
has revealed novel architectural features of the genome. For
example, we have found that the E. coli chromosome contains
hundreds of kilo-base scale regions, bound by nucleoid proteins,
that, through their transcriptional silencing effect, appear to
function as prokaryotic analogs of eukaryotic heterochromatin.
For decades, the dominant approach to
quantifying protein abundance and interactions has been
mass-spectrometry (MS). However, MS-based approaches lack the
affordability, scalability, and standardization required for routine
comprehensive profiling of proteomes at a scale required for
advances we envision. With support from an NIH Transformative award,
we have developed an alternative approach to proteomics based on
attachment of proteins to their encoding mRNA sequences in vivo.
This technology, we call In vivo mRNA-display enables us to
query abundance, interactions, and localization of proteins through
identification of their accompanying nucleic-acid tags (Oikonomou et al., PNAS,
2020).
In
this way, we recast proteomic analysis as a DNA sequencing problem,
bypassing mass-spectrometry bottlenecks, and setting proteomics on
the path to benefit from exponentially improving cost and throughput
of next-generation sequencing. Furthermore, by preserving the
intrinsic post-translational modification states of proteins, in
vivo mRNA-display enables more accurate representation of
native proteomes, critical for precise inference of protein function
and interactions. We are using in vivo mRNA display as the
platform to scale a variety of proteomic technologies to enable
routine generation of dynamic global maps of protein function and
interactions. We are also developing in vivo mRNA display as
a versatile foundation for protein engineering and synthetic biology
applications.
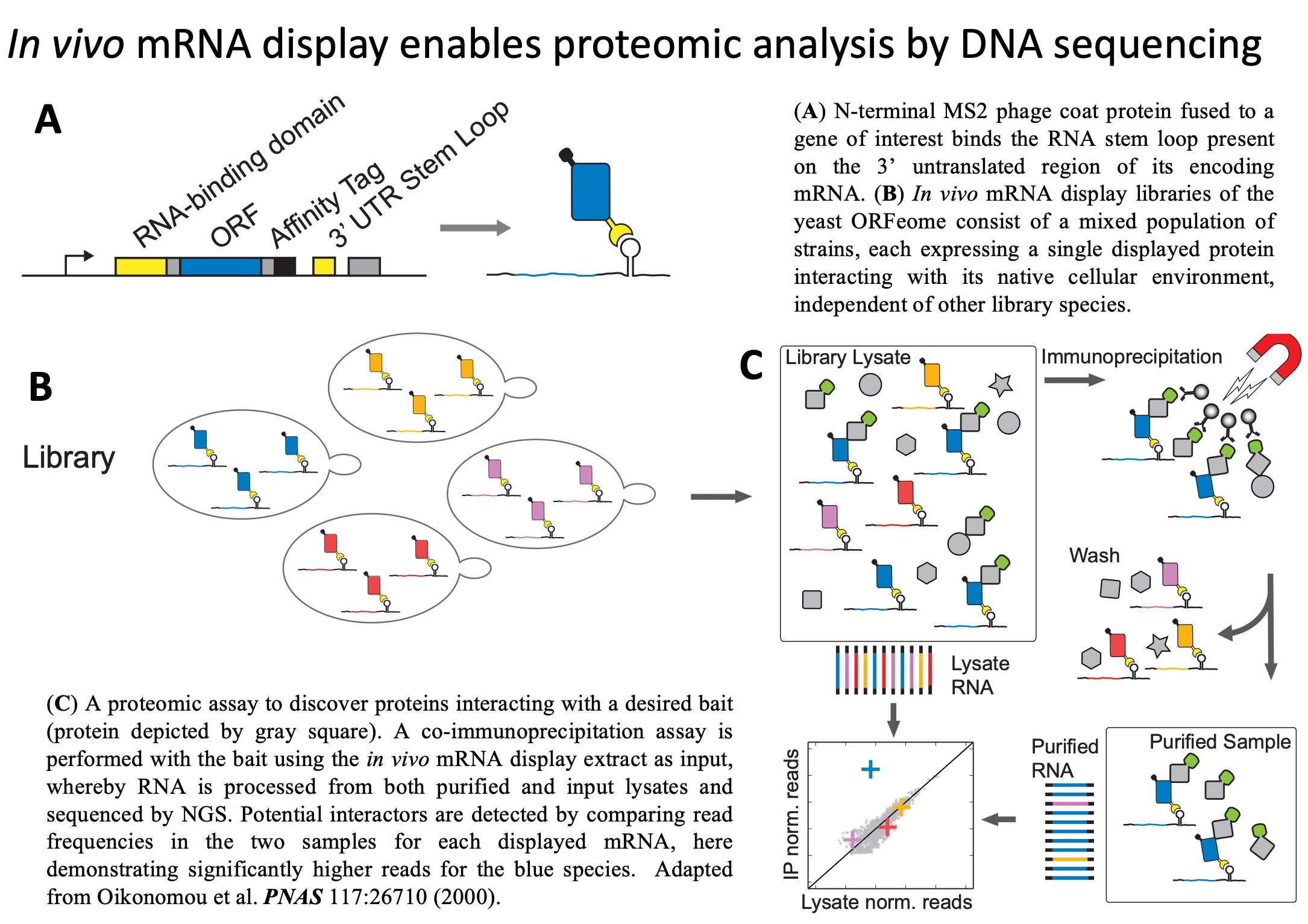
Areas of active
investigation include:
-Cellular adaptation through stochastic tuning of gene expression
-Genetic basis of antibiotic sensitivity, resistance, and
persistence
-Microbial adaptation to extreme environments
-Predictive models of transcriptional, post-transcriptional, and
translational regulatory networks
-Reverse engineering regulatory networks in cancer and metastasis
-Regulatory networks of cellular and organismal aging
-DNA-sequencing based next-generation proteomics technologies
-Deep mapping of molecular interactions in networks of proteins,
DNA, RNA, and metabolites
-CRISPR-based functional genomic technologies
-Microbiome functional genomics
-Synthetic transcriptomic and proteomic technologies for cellular
engineering
Join our team:
We are looking for creative and ambitious people to join our highly interdisciplinary and interactive group. Please send inquiries to: st2744 [at] columbia.edu